Detecting narrativity across long time scales
Our lab has a new article out that will be appearing in the Computational Humanities Research Conference Proceedings (CHR 2021).
In this project, we develop computational methods for measuring the degree of narrativity in over 335,000 text passages distributed across two- to three-hundred years of history and four separate discursive domains (fiction, non-fiction, science, and poetry). As you can see in the figure below, we show how these domains are strongly differentiated according to their degree of narrative communication and, second, how truth-based discourse has declined considerably in its utilization of narrative communication.
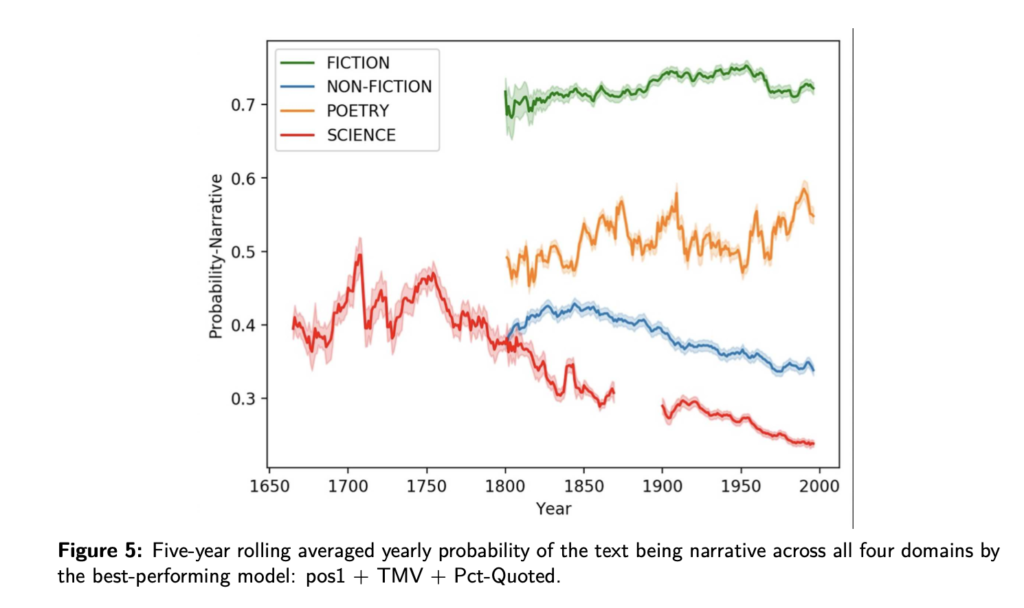
We think this long term historical de-narrativization of science has important implications for future efforts that aim to address public trust in science. We know that narrative is extremely important for the way people process information, believe information, and form trust. Re-narrativizing scientific findings thus has a steep hill to climb to reverse this historical trend. But it is one we think will be essential as we move forward as a society.
Beyond the particular findings, we found working on detecting narrative to be particularly fun and interesting as a problem for machine learning. While a wealth of recent work in the field of natural language processing has engaged with the detection of different dimensions of narrativity, no work to our knowledge has undertaken the more elementary task of narrativity detection itself. Can we reliably predict whether a span of text is engaging in narrative communication and if so, to what degree of intensity?
To do so we spent weeks meeting and discussing individual passages to better understand how narrative communication works and how we could achieve consensus about its presence and degree of intensity. And then we figured out ways to see if a machine could approximate our judgments (see next figure). This project is a really nice example of working across a variety of divisions — different student levels and different disciplinary expertises. Undergrads in the humanities worked alongside grad students in computer science to develop these models and I was an active participant during the entire process as well.
While I’ve been saying this for a while it bears repeating: to understand how automated language systems work, close reading is essential. Engaging deeply with the nature of texts is at the core of humanistic machine learning.
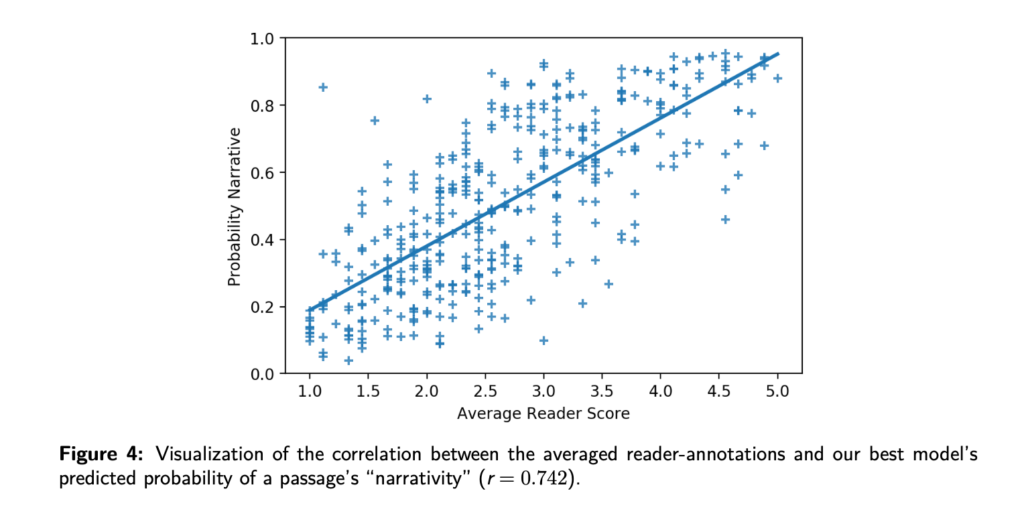